By Alessandra Vescio
Since its first appearance in November 2022, the artificial intelligence chatbot ChatGPT developed by OpenAI has fascinated and captivated many for its capability to create human-like content in a way that cannot be compared to anything else we have seen before. People started testing ChatGPT and playing with it, asking questions and sharing its almost perfect or sometimes even funny answers. But more than funny answers, generative artificial intelligence, a term used to describe the algorithms capable of creating new content, represents a potential disruptive change.
ChatGPT has been run on a technology known as GPT-3.5, now upgraded to GPT-4, which has been described by OpenAI as “large multimodal model […] that, while less capable than humans in many real-world scenarios, exhibits human-level performance on various professional and academic benchmarks”. ChatGPT can answer questions on a range of topics and the new upgrade allows it to handle and discuss visual information although it cannot create images as platforms such as Midjourney or OpenAI’s DALL-E can.
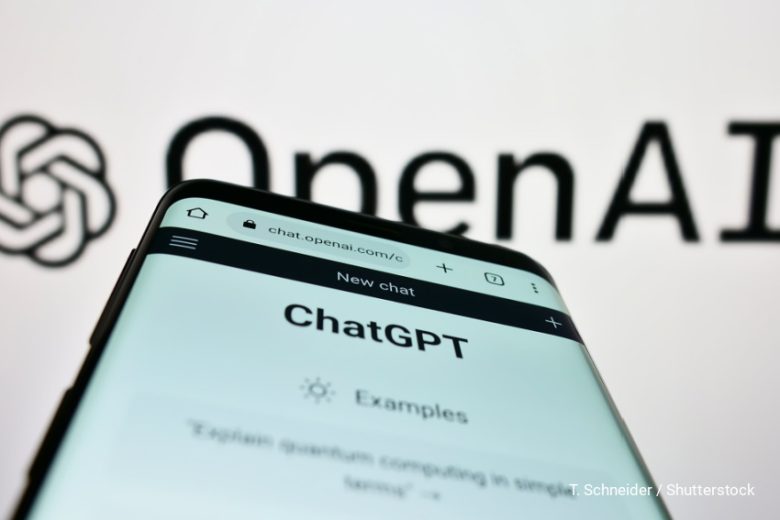
On the other hand, ChatGPT can mimic authors’ writing styles and produce essays and short novels. For instance, it appeared as the author or co-author of the more than 200 e-books available in Amazon’s Kindle Store in February.
However, the way generative AI works and its impact on journalism and society must be addressed, not only from the point of view of possible benefits but also its risks.
Copyright
In order to achieve their capabilities, systems like ChatGPT have been trained on a large amount of content available on the Internet, which prompts questions about the concept of copyright. Although AI researchers, especially in the US, often invoke the legal provision of ‘fair use’, which allows the use of copyrighted material without permission under limited conditions, artists, authors and media organisations have pointed out that these systems use their creativity and work without respecting their rights and/or paying them.
Journalist Alex Kantrowitz said a post of his newsletter ‘Big Technology’ was plagiarised and went viral, while Isabelle Doran, head of the Association Of Photographers (AOP), which promotes and protects the rights of photographers in the UK, reported: “We have clear evidence that image datasets, which form the basis of these commercial AI generative image content programs, consist of millions of images from public-facing websites taken without permission or payment”, leaving AOP’s members in “shock” and worried about their intellectual property and its value.
The problem is not only about copying their content: generative content also becomes a strong competitor on the Internet of the material it has exploited to be produced. For instance, publishers are concerned that generative content not only uses their work freely, but also drives traffic away from their platforms – and thus brings less money to publishers’ websites – by not providing any links to the sources used and giving very comprehensive answers. This is the case with Bing. As Microsoft CEO Satya Nadella said “On Bing Chat, I don’t think people recognise this, but everything is clickable”, but the answers given by the chatbot are described as so detailed that clicking on a link is neither really necessary nor encouraged.
To try to overcome these problems and still take advantage of artificial intelligence, the American provider of stock photographs and videos Shutterstock announced a partnership with OpenAI and a new tool capable of creating images based on text prompts. It also announced “a fund to compensate artists” who “have contributed to develop the AI models,” but left much doubt as to whether this compensation will ever be sufficient.
Diversity
The issues raised by generative artificial intelligence do not stop there. As many experts have pointed out, there is a high risk of reproducing prejudices and bias while being perceived as neutral. For instance, AI image generators were studied and found to amplify stereotypes, e.g. by reproducing a ‘white ideal’ when asked to create an image of an attractive person, or a brown face with dark hair and beard as a result of ‘a terrorist’. Similarly, sexist and racist answers were given by ChatGPT.
OpenAI has put some guardrails to “decline inappropriate requests”, and Sam Altman, OpenAI CEO, admitted “ChatGPT has shortcomings around bias” and that they are “working to improve it”, but much more needs to be done in this field. “Generative AI models are trained on both helpful and toxic data”, data journalist and author of ‘More Than a Glitch: Confronting Race, Gender, and Ability Bias in Tech’ Meredith Broussard told MDI. “The entire Reddit corpus, for example, is part of the training data for ChatGPT. Parts of Reddit are delightful, parts are not. Which part does ChatGPT rely on? All of it,” she explained.
“Size doesn’t guarantee diversity”, Timnit Gebru, computer scientist and founder and executive director of the Distributed Artificial Intelligence Research Institute, said.
Gebru worked as co-head of Google’s Ethical AI research team, from which she said she was fired after raising social and ethical issues of some AI systems in a paper she co-authored. Talking about generative artificial intelligence, Gebru said:
“There are so many different ways in which people on the Internet are bullied off the Internet. We know women get harassed online all the time, we know people in underrepresented groups get harassed and bullied online.”
And when people are bullied in a space, they leave that space, therefore
“The text that you’re using from the Internet to train these models is going to be encoding the people who remain online, who are not bullied off—all of the sexist and racist things that are on the Internet, all of the hegemonic views that are on the Internet. So, we were not surprised to see racist, and sexist, and homophobic, and ableist, etcetera, outputs,” Gebru explained.
A solution cannot be found while there is indiscriminate use of the data and content available online. Meredith Broussard told MDI:
“There is an old saying in computing: garbage in, garbage out. We won’t see forward progress on diversity by using models that are trained on problematic data.”
This is also why an ethical framework and regulation are needed.
Subramaniam (Subbu) Vincent, director at the Journalism and Media Ethics program at Markkula Center for Applied Ethics, Santa Clara University, told MDI:
“Part of the problem is how business elites are getting away with framing innovation as stifled by regulation or fundamental ethical constraints. There is a more ethical framing for the word ‘innovation’. Harm-reducing regulations or other structural constraints can themselves spur novel innovations that benefit wider groups of people and communities. There is a history of this too. Ignoring this history is also a risk.”
Ethics, journalism and risks
The race to produce generative artificial intelligence is a focus of large tech companies. For example, Microsoft announced the extension of its long-term partnership with OpenAI through a “multiyear, multibillion dollar investment” in its technology and the inclusion of a chatbot based on OpenAI’s GPT-4 on Bing and Microsoft365. The Chinese search engine Baidu has started work on a similar product, while Google has accelerated research and work on its own AI-powered chatbot called Bard.
Generative AI “is a major revolution at the level of oil for transportation and fuel, plastics for everyday life, computers for automation, and so forth,” Subbu Vincent told MDI, but the main problem is that “we cannot fully anticipate all the risks and potential problems downstream of where we are today, if we don’t look at history,” and “we are still not. The big AI companies are releasing the technology in a competitive race for adoption and returns, which seems legal, but weakly regulated.”
Among the consequences of weak regulation of generative artificial intelligence could be an overproduction of content with “no commitment to the truth”. The two photos of Trump being arrested and the Pope wearing a white puffer jacket were not unanimously understood to be fake images, but as the director of the Tow Center for Digital Journalism at Columbia University’s Graduate School of Journalism Emily Bell explained, the main risk goes beyond easily unmasked examples, and rather occurs “with material that overwhelms the truth or at least drowns out more balanced perspectives”.
First of all, generated AI is unable to distinguish between fiction and truth and using them as learning tools is dangerous. Moreover, when used in newsrooms, the review carried out by editors is no guarantee of accuracy, as Vincent explained. And, as social media have shown in recent years, an uncontrolled and unfiltered amount of news and the circulation of fake news have a huge impact on societies and a detrimental effect on democracies.
However, although generated content on websites is nothing new – “Generated sports stories have been going on for a long time already,” Subbu Vincent explained to MDI – newsrooms are increasing their use of these systems, and the current economic crisis is likely to push this trend since it is cheaper using AI than paying journalists.
Buzzfeed has announced it would start using AI for its quizzes, which return customised answers to users, but Futurism reported that AI-generated articles, specifically travel guides, have been published on the website. A Buzzfeed spokesperson said they are “experimenting a new format” and that human editors are involved in the process.
“More machine generation will likely happen in story areas where a human editor can still catch and fix factual errors and obvious stereotyping,” Vincent said, as well as a new reorganization of newsrooms.
On the other hand, Vincent believes that
“the most important distinction between Large Language Models based AI (generative AI) and journalistic work is that some types of storytelling require humans on the ground. Covering present and imminent realities as they unfold involves anticipating powerful people trying to stop stories from getting to facts, documents, and truths. So the stories that get unshackled from deep within bureaucracies, public affairs require serious journalistic talent and review. LLMs are trained with cutoff dates so their text generation capacity is mostly to mimic what humans might say in a given set of situations. That is not journalism.”
Journalism indeed “will always have value for the public and communities if journalists stay with storytelling forms, genres, and formats that require multiple interrogations of what is happening in the present,” Vincent said, “or if stories” are used “to connect the present with the past, so that history is contextualized as opposed to selectively shining past glories,” and if journalists “actively think and decide the framing and complicate the narratives for stories away from simplistic binaries.”
Moreover, journalists can benefit from generative AI as well, by delegating time-consuming tasks to technologies. On the other hand, newsrooms that decide to create content using AI, “must develop a ‘Generative AI ethics and disclosure policy’,” Vincent said.
“This must be easily accessible and tell readers and viewers when, where, why, and how a newsroom uses generative AI. Asking readers to guess, or keeping the processes subtle and invisible is both unethical and is missing out on an opportunity to educate people.”
Photo Credits: T. Schneider / Shutterstock